GPU Dedicated servers are specialized servers that are equipped with a graphics processing unit. These servers are proficient in parallel processing, which allows the process to run the model faster than on CPU-based systems. It is the best GPU dedicated server that offers to increase power and speed for running devices such as data analytics, artificial intelligence, and machine learning. The GPU Dedicated Server is one of the most important servers that uses graphics processing units for some part of the mathematical calculation. It can process the various pieces of data and make them useful for machine learning, gaming applications, and video editing. The future of GPU Dedicated Server in AI and machine learning is going in the direction of substantial growth and evolution. It will become very useful in the future because it is used to handle complicated mathematical and geometrical calculations in very little time. That’s why we can say that GPU Dedicated servers are best for AI and machine learning.
Difference Between CPU and GPU Server: A Comparative Analysis
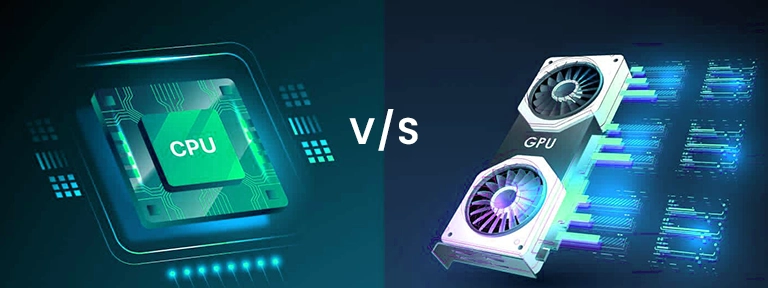
The main difference between CPU and GPU servers is that CPUs can handle AI tasks, but they are not efficient for tasks that require high parallelism. It is useful for tasks that require sequential algorithms or perform complex statistical calculations. The GPU Dedicated Server is optimized for parallel processing, and its architecture is designed to handle multiple operations simultaneously. It handles mathematical operations in significantly less time.
Benefits of GPU Servers in AI and Machine Learning
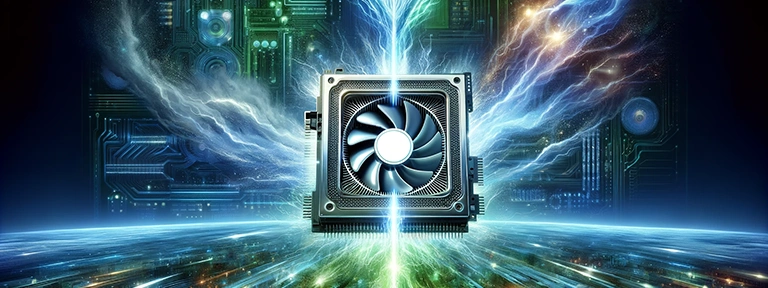
As we all know that the future of GPU Dedicated Server is most profitable on AI and Machine Learning. There are several benefits of GPU Servers which define that the value AI and Machine Learning is very necessary in the GPU Servers:
Parallel Processing: GPUs have more than thousands of cores for parallel execution, which makes them ideal for handling the mathematical and vector operations that are common in AI and machine learning algorithms.
Fast Training: Training the deep learning modules in which large numbers of computational datasets are involved. It takes less time to train these models in comparison to CPU-based systems.
Handle Large Data Sets: GPU Servers manage and execute large volumes of computational datasets simultaneously, which is most important for game applications, AI, machine learning, and data analytics.
Grow the performance for inference: GPU Server is the most powerful tool that maintains the speed of the data pipeline with a deep neural network. The deep neural network inference runs up to 10 times faster on the GPU server as compared with the CPU.
How GPU servers are transforming AI and ML applications
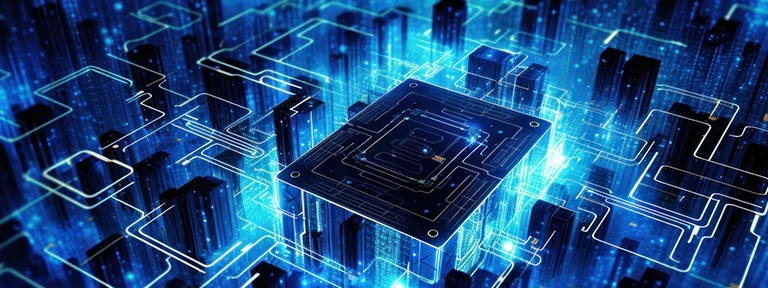
GPU servers is recognized as the best GPU server for machine learning that transforms AI and machine learning applications for better results and calculations. They specialize in designing the hardware for processing large blocks of data simultaneously and transforming it into machine learning, video processing, and accelerating complex mathematical calculations. Our best Dedicated GPU servers offer high cost efficiency for AI and machine learning, and their ability to speed up processing depends on the specific implementation and nature of the data. There are various key points of transforming AI and ML applications in which GPU servers make an impact on AI and ML applications.
- Parallel Processing and Large Scale Data Handling: GPU Dedicated servers optimise the data for parallel processing, making them ideal for the parallel processing that is required in AI and ML applications. It can manage and process the data on a very large scale, which is essential for training deep learning models.
- Increased the model Performance: The GPU parallelism allows for higher throughput of data processing, trains the model on larger datasets, and improves the model iteration and cycles, resulting in better accuracy of the results.
- Cost Efficient: GPUs offer more cost efficiency for the AI and machine learning tasks that can take advantage of their parallel processing capabilities. By applying the power of the CPU servers, organisations can achieve the highest throughput, which reduces the GPU Server cost of hardware and energy.
- Support for Advanced Algorithms: GPU servers support the deep learning algorithms, which require substantial computational power. They are used for high-frequency algorithms where speed and efficiency are paramount. AI and ML applications are more efficient after training, and the inference process is rapid. GPU servers have appropriate deep learning frameworks such as TensorFlow, PyTorch, and Keras, which handle matrix multiplication and other operations.
- Real Time Processing and Interactive AI : GPU Server works on various real-time applications such as deep learning, artificial intelligence, scientific computing, and data analytics. Once the AI model is trained, it needs to be deployed for inference tasks such as real-time processing of data. GPU servers have the capability to perform inference tasks with low latency and make them suitable for real-time applications like autonomous vehicles, speech recognition, and recommendation systems.
- Innovation and Development: GPU servers have become experts in modern computing due to their ability to handle parallel processing tasks efficiently. Their innovation and development perform in several key areas, such as AI, ML, data analytics, and high-performance computing. The GPU Servers run on a wide range of applications, making them indispensable in many industries. The role of GPU servers is critical after it transforms into AI and ML applications.
Why GPUs are essential for AI, ML and high-performance computing
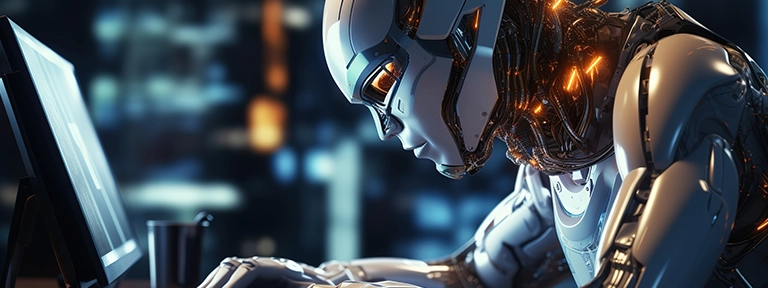
GPU Dedicated Servers, are recognized as Best GPU Dedicated Server, are most necessary for AI, Training complex AI models and performing large-scale data analysis, and machine learning. There are a number of reasons why a GPU dedicated server is essential for AI and ML. These reasons are mentioned below.
- Parallel Processing Capabilities: GPU servers are designed to handle thousands of simultaneous threads, which can handle the tasks in parallel processing and handle all the matrix multiplications.
- CUDA and Other Frameworks: NVIDIA’s CUDA is known as Compute Unified Device Architecture and enables developers to write programs that can exploit the parallel processing power of GPUs.
- Performance: GPUs can perform multiple calculations more quickly than CPUs. In the GPU architecture, GpU Server is optimised for handling multiple operations in parallel. High throughput in GPUs is the ability to train AI models and run simulations.
- Energy Efficiency: GPUs energy efficiency is higher compared to CPUs in the various AI and HPC tasks. They can deliver higher performance per watt. The cost efficiency is much lower than that of the CPU server. It is a cost-saving process.
- Specialised Hardware: Modern GPUs come with special hardware, such as Tensor Cores, which are specialised to be optimised for deep learning operations, such as tensor operations used in neural networks.
- Ecosystem and Software Support: A vast ecosystem of libraries and frameworks is optimised in the GPU Server, and it makes the work easy for developers who work for AI, HPC, and ML.
- Multi-GPU Systems: GPU Dedicated servers are used as a parallel processing unit across multiple devices. They scale up the computing power for AI, ML, and HPC training applications. This scalability is needed to handle large numbers of calculations and datasets.
- Real-World Applications: In this process, training on the deep learning AI models includes large amounts of data and computations, which are accelerated by the GPU servers. It also improves the performance of AI inference. In HPC, GPUs handle the complex calculations and data processing tasks in fields such as physics, chemistry, and climate science.
Conclusion:
GPU Dedicated servers recognized as best GPU Dedicated Server is the most crucial part for AI and ML in the future due to their high computational process, parallel processing capabilities, high performance, efficiency, specialized hardware, robust software support, and scalability. In conclusion, we can only say that the GPU Dedicated server is the Best dedicated gpu server in real-world technology, and the future of the GPU server depends on AI, machine learning, and other real-time applications such as deep learning, artificial intelligence, scientific computing, and data analytics. So, we accept and embrace this new technology, which is very important for GPU Dedicated servers in the future.
Read More : Comparing Leading GPU Server Providers: Which One is Right for You?